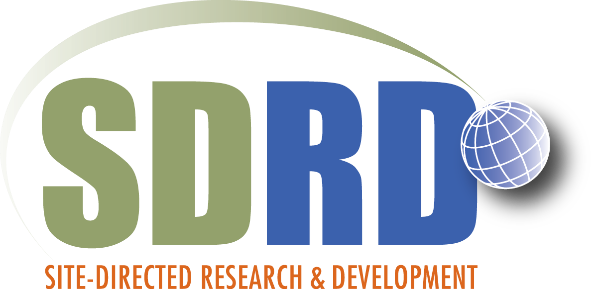
Measurements for Combined Gamma-Ray and Video Modalities
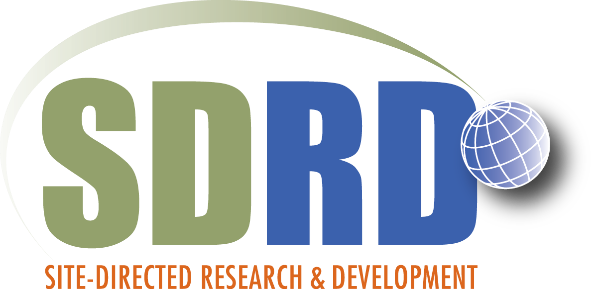
Project #: 22-022 | Year 1 of 3
Paul Mendoza,a Chris Burt,a Roy Abbott,a Cameron Priest,a Shreyas Kamath,a Kevin Lee,a Johanna Turk,a Rusty Trainham,a Michael Willis,b Lauren Overbay,c William Fordc
aSpecial Technologies Laboratory; bOak Ridge National Laboratory (ORNL); cLos Alamos National Laboratory
Executive Summary
The Nevada National Security Site (NNSS) has previously created a network of spectral gamma sensors in Northern Virginia (NoVA) supporting Defense Nuclear Nonproliferation (DNN) Research and Development (R&D). The array of sensors historically generated gamma data used in after-the-fact gamma anomaly detection and source identification algorithm development. In year one of this three-year SDRD, the array hardware was upgraded to incorporate video data collection and edge computation capabilities. These changes specifically aim to extend NoVArray’s applicability space from dataset generation to real-time information production through strategic use of newly added computational resources. We have completed two prototype units and begun to optimize our software for deployment on embedded systems. In year two of this effort, we will finalize our software and begin initial hardware testing at ORNL with relevant gamma sources. We will also begin to upgrade the 18 NoVArray units currently deployed in Northern Virginia.
Description
Sensor systems designed to identify gamma sources date back at least 70 years, providing the benefit of assessing radioactive composition of unknown materials. Initial advancements focused on detector materials, energy resolution, data collection, software for analysis, etc. Although new solutions to these areas are still forthcoming, technology maturation has allowed effective sensor systems to be deployed in a wide variety of contexts, from radioactive source identification in controlled laboratory environments to wildly dynamic border crossings. Every identification from past sensor deployment must make assumptions about measurement conditions (collection time, orientation, estimated shielding, material types, stationarity, etc.). Advancements in the field are not only marked by hardware (with supporting software) improvements, but also by the algorithmic improvements necessary for deployment in different contexts with fewer measurement condition assumptions.
This project hopes to help advance the field beyond the shielding, collection time, and stationarity assumptions by providing real-world training data for machine learning (ML) algorithms that relate to the data in a way that is not human-centric. Additionally, the project aims at incorporating the trained algorithms into edge calculations for real-time source identification with complex gamma data. Other recent works have focused on source localization, but have struggled with identification (Marshall et al. 2020, Liang and Talley 2021, Osthus et al. 2022). This project builds on recent success in source identification with mobile sources (Moore et al. 2020, Hoteling et al. 2021).
Data generation, collection, hardware, and computer requirements need to be addressed concurrently so that analysis and data generation occur continuously at each node. This is accomplished by aggregating relevant data and designing a system that meets the requirements. Gamma efforts focus on a convolutional neural network (CNN) architecture for source identification (Moore et al. 2020) and an information-based anomaly detection (Hoteling et al. 2021) codebase to detect measurement times of interest. Detector characterization and calibration follows standard gamma practice (Gilmore 2008, Knoll 2010). Video data is processed through a custom object recognition algorithm, leveraging the popular open source algorithm, You Only Look Once (Redmon et al. 2015). Mobile detector data will be generated by injecting Gamma Detector Response and Analysis Software (GADRAS) (Mitchell and Mattingly 2019) source simulations into background mobile data with random sampling.
Conclusion
In year one of this SDRD project, we updated hardware design (implemented in two prototype nodes and one test node), added contextual video sensor measurement and analysis capability, and began to implement real-time data collection and processing. Legacy NNSS data analysis algorithms and database architecture were investigated and provided a basis for software implementation, including a novel gamma detection and isotope identification technique that utilizes CNNs. Year two of this project will finalize software architecture as well as deploy the hardware and software upgrades to nodes at ORNL for testing with real-world gamma sources. We will begin the upgrade process for the 18 legacy systems in Northern Virginia, by removing them from their current deployed locations, and shipping back to Santa Barbara for hardware modifications.
Mission Benefit
This platform is ideal for the NNSS because it plays to the already recognized strengths of providing data, performing field measurements, and truthing remote collections. NoVArray is an existing asset owned by the NNSS, which has proved to be a popular source of data for the research community. Nearly every lab working on ML for gamma detection recently has published papers or given presentations citing their research on this dataset. By continuing to invest in meaningful upgrades, the NNSS can leverage a known asset to encourage future collaboration and provide a fresh hook for the inclusion of the NNSS in multi-lab funding proposals.
DNN R&D has demonstrated interest in these sorts of multimodal platforms (Canary, Merlyn, EMU), and we anticipate future attention from state and local governments, including our current collaborators in Northern Virginia and Los Angeles. Modifications to this platform allow us to be the provider of a significant dataset that has already drawn wide interest from academia, national laboratories, and industry. This is a low risk opportunity to build important collaborations with other labs in the data analytics space in order to help us stay relevant in a ML/AI ‘marketplace.’
References
Gilmore, G. 2008. Practical Gamma-Ray Spectroscopy. 2nd ed. West Sussex: John Wiley & Sons.
Hoteling, N., E. T. Moore, W. P. Ford, T. McCullough, L. McLean. 2021. “An Analysis of Gamma-Ray Data Collected at Traffic Intersections in Northern Virginia.” arXiv. 2104.04137 [physics.soc-ph]. https://doi.org/10.48550/arXiv.2104.04137.
Knoll, G. F. 2010.Radiation Detection and Measurement. 4th ed. Hoboken: John Wiley & Sons.
Liang, J. F., K. Talley. 2021. “Enhanced Directional Detection of Gamma Sources.” Nucl Instrum Methods Phys Res A.1002: 165304. https://doi.org/10.1016/j.nima.2021.165304.
Marshall, M. R., et al. 2020. “3-D Object Tracking in Panoramic Video and LiDAR for Radiological Source–Object Attribution and Improved Source Detection.” IEEE Trans Nucl Sci.68(2): 189-202. doi: 10.1109/TNS.2020.3047646.
Mitchell, D., J. Mattingly. 2009. Gamma Detector Response and Analysis Software (GADRAS) v. 16.0. 001744IBMPC01. Computer software. Albuquerque, New Mexico, and Livermore, California: Sandia National Laboratories.
Moore, E. T., J. L. Turk, W. P. Ford, N. J. Hoteling, L. S. McLean. 2020. “Transfer Learning in Automated Gamma Spectral Identification.” arXiv. 2003.10524v1[physics.data-an]. https://doi.org/10.48550/arXiv.2003.10524.
Osthus, D., et al. 2022. “Tracking the Location of a Road-Constrained Radioactive Source with a Network of Detectors.” Nucl Instrum Methods Phys Res A.1039: 166992. https://doi.org/10.1016/j.nima.2022.166992.
Redmon, J., S. Divvala, R. Girshick, A. Farhadi. 2015. “You Only Look Once: Unified, Real-Time Object Detection.” arXiv. 1506: 02640v5[cs.CV]. https://doi.org/10.48550/arXiv.1506.02640.
This work was done by Mission Support and Test Services, LLC, under Contract No. DE-NA0003624 with the U.S. Department of Energy. DOE/NV/03624–1658.
Return to Enabling Technologies for Autonomous Systems and Sensing
Go to SDRD Annual Report Index
Return to SDRD Homepage